Yingxu Wang, PhD, FWIF, FICIC, SM IEEE, SM ACM, President ICIC
PhD The Nottingham Trent University, UK President, ICIC; Professor of Brain Scence at HBI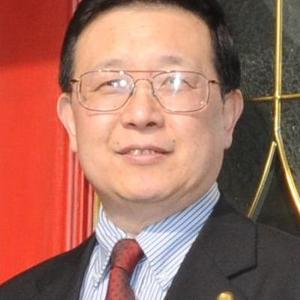
Areas of Research
Dr. Wang and his lab’s research interests across 10+ disciplines encompassing intelligence science, brain science, computer science/engineering, software science/engineering, denotational mathematics, robotics, neural science, cognitive linguistics, system science, knowledge science, and data science. A series of basic studies and innovative projects have led to 480+ publications (36 invited keynotes speeches, 180+ journal papers, 260+ conference papers, and 29 books/proceedings). According to Research Gate, he is a top 2.5% scholar worldwide and top 10 at U of C (https://www.researchgate.net/profile/Yingxu_Wang).
Cognitive robots (CRs) are a cutting-edge technology of autonomous robots that is capable of learning and reasoning mimicking the brain.
Cognitive Informatics (CI) is a transdisciplinary field that studies the internal information processing mechanisms of the brain, the underlying abstract intelligence (aI) theories and denotational mathematics, and their engineering applications in cognitive computing, computational intelligence, and cognitive systems. Cognitive Computing (CC) is a cutting-edge paradigm of intelligent computing methodologies and systems based on cognitive informatics, which implements computational intelligence by autonomous inferences and perceptions mimicking the mechanisms of the brain.
Learning is a central ability of machine intelligence and cognitive systems for knowledge and behavior acquisition. The taxonomy of machine learning methodologies can be classified into six categories known as object identification, cluster classification, pattern recognition, functional regression, and behavior (game) generation, and knowledge acquisition. The current project focuses on the 6th category of cognitive knowledge learning.
Denotational mathematics (DM), as a supplement to classic analytic mathematics defined in R, is a collection of novel mathematical structures that formalize rigorous expressions and operations on complex entities known as hyper-structures (H) beyond R. Instances of H are such as formal concepts, relations, knowledge, behavioral processes, intelligence, and systems. Paradigms of DM developed in my lab are such as concept algebra, system algebra, semantic algebra, inference algebra, fuzzy probability algebra, and big data algebra. This leads to the emergence of mathematical engineering (ME) that studies formal structural models and functions of complex abstract and mental objects and their systematic and rigorous manipulations.
A resent finding in my lab reveals that big data systems in nature are a recursive n-dimensional typed hyperstructure (RNDTHS). This topological property of big data indicates that the mathematical foundation of big data science is underpinned by a denotational mathematical structure known as Big Data Algebra (BDA) [Wang, 2015], which is an efficient mathematical means for dealing with the inherited complexities and unprecedented challenges in big data engineering.
The contemporary wonder of sciences and engineering has recently refocused on the starting point of them: how the brain processes internal and external information autonomously rather than imperatively as those of conventional computers? Brain science is both a classical and contemporary discipline that studies the functional and logical model of the brain, a set of cognitive processes of the mind, internal information processing mechanisms, and their engineering applications towards brain-inspired systems. A key notion in brain science is that the brain and natural intelligence may only be explained by a hierarchical and reductive theory that maps the brain through the embodied neurological, physiological, cognitive, and logical levels from bottom-up induction and top-down deduction. An abstract intelligence theory has been created for modeling the structures and functions of the brain across these hierarchical levels. A Layered Reference Model of the Brain (LRMB) [Wang et al., 2006] has been developed in order to explain the functional mechanisms and cognitive processes of the brain. A Cognitive Functional Model of the Brain (CFMB) [Wang, 2012] has been developed, which maps the natural intelligence functions of the brain onto its neural and physiological structures. These theoretical and transdisciplinary models provide a blueprint for a wide range of applications in the development of highly intelligent systems such as cognitive computers, cognitive knowledge search engines, autonomous learning machines, and cognitive robots.
Intelligence is a mental process that transfers information into behaviors and knowledge. Intelligence is the highest level of cognitive ability of human brains and artificial counterparts. Intelligence science is a contemporary discipline that explores the nature of intelligence and its biological, neurological, physiological, functional, logical, mathematical foundations. It reveals how intelligence is aggregated among the cognitive objects of data, information, knowledge, and wisdom from the bottom up. My lab focuses on the abstract intelligence theory of intelligence science, which is a formal enquiry of both natural and artificial intelligence theories and underpinned mathematical models. The abstract intelligence theory provides a theoretical foundation for integrating brain informatics, cognitive informatics, neuroinformatics, and bioinformatics. Cognitive engineering of intelligence science is applied in a wide range of fields such as natural, artificial, machinable, computational intelligence.
Software science [Wang, 2007, 2014] is a discipline that studies the formal properties and mathematical models of software, general methodologies for rigorous and efficient software development, and coherent theories and laws underpinning software behaviors and software engineering practices. This project focuses on the general mathematical model of software and systems. It reveals that any software system is a Cartesian product between the functional behaviors and abstract data entities formally described as the sets of process models (PMs) and structure models (SMs), respectively.
Supervising degrees
More information
Working with this supervisor
New PhD students are welcome on exploratory and innovative projects such as: a) Cognitive robotics; b) Deep machine learning; c) Cognitive systems; d) Software science theories; and e) Industry-sponsored projects. Both theoretical (mathematical) and experimental (MATLAB/programming) skills are expected for strong candidates.
Contact this supervisor
Complete the following form if you are interested in working with this supervisor for your Graduate Program. All fields are required, unless indicated otherwise.
Collection of personal information
Your personal information is collected under
the authority of section 33(c) of the Freedom of Information and Protection of Privacy Act. If
you have any questions about the collection or use of this information, please visit our
Access to Information page.